
Automotive Case Study
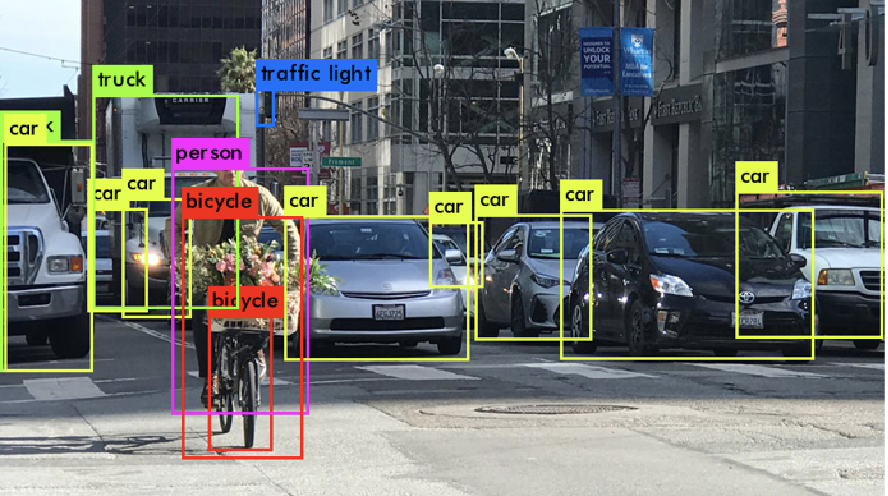
In recent years, there has been a remarkable surge in interest and excitement surrounding self-driving cars. With rapid advancements in artificial intelligence (AI) and autonomous vehicle technology, self-driving cars hold the potential to revolutionize transportation, offering numerous benefits such as improved road safety, reduced traffic congestion, and enhanced mobility for individuals who are unable to drive.
In the context of self-driving cars, explainability entails providing detailed explanations for the AI system’s decision-making, including the factors considered, the rationale behind specific actions, and the source of data used for decision-making. This transparency allows regulatory bodies, engineers, passengers, and other stakeholders to comprehend how the AI system operates, its limitations, and potential vulnerabilities.
Explainability is essential for deploying AI systems safely and for building trust in AI systems, which will allow for their widespread adoption.
Overall, safety certification and explainability requirements for critical
AI systems in self-driving cars serve to:
Challenge: The deployment of self-driving cars in the real world relies heavily on advanced artificial intelligence AI systems that handle critical tasks such as perception, decision-making, and control. Given the potential risks associated with autonomous vehicles, ensuring safety and explainability becomes paramount. However, current AI systems lack the reliability, explainability and traceability required for ensuring safety standards for Critical autonomous AI-Based Systems (CAIS).
A key focus of this project is to design detection systems that adhere to both safety and explainability requirements. These requirements ensure that AI systems meet rigorous safety standards and provide transparent explanations for their actions and allow for the deployment of self-driving cars to proceed with greater confidence and reliability
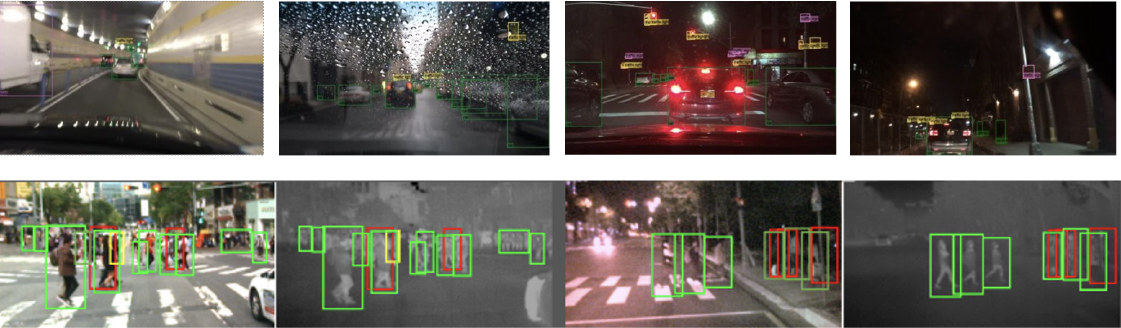
Figure 2: Detection of road users to inform timely decisions
The SAFEXPLAIN automotive case study develops advanced methods and procedures that enable self-driving cars to accurately detect road users, estimate their distance from the vehicle, and predict their trajectories.
These capabilities are vital for making timely decisions and executing appropriate collision avoidance maneuvers. By incorporating this technology, self-driving cars can navigate complex traffic scenarios while ensuring the safety of pedestrians, cyclists, and other vulnerable road users.
Read about our progress in the automotive case study: