SAFEXPLAIN: From Vision to Reality
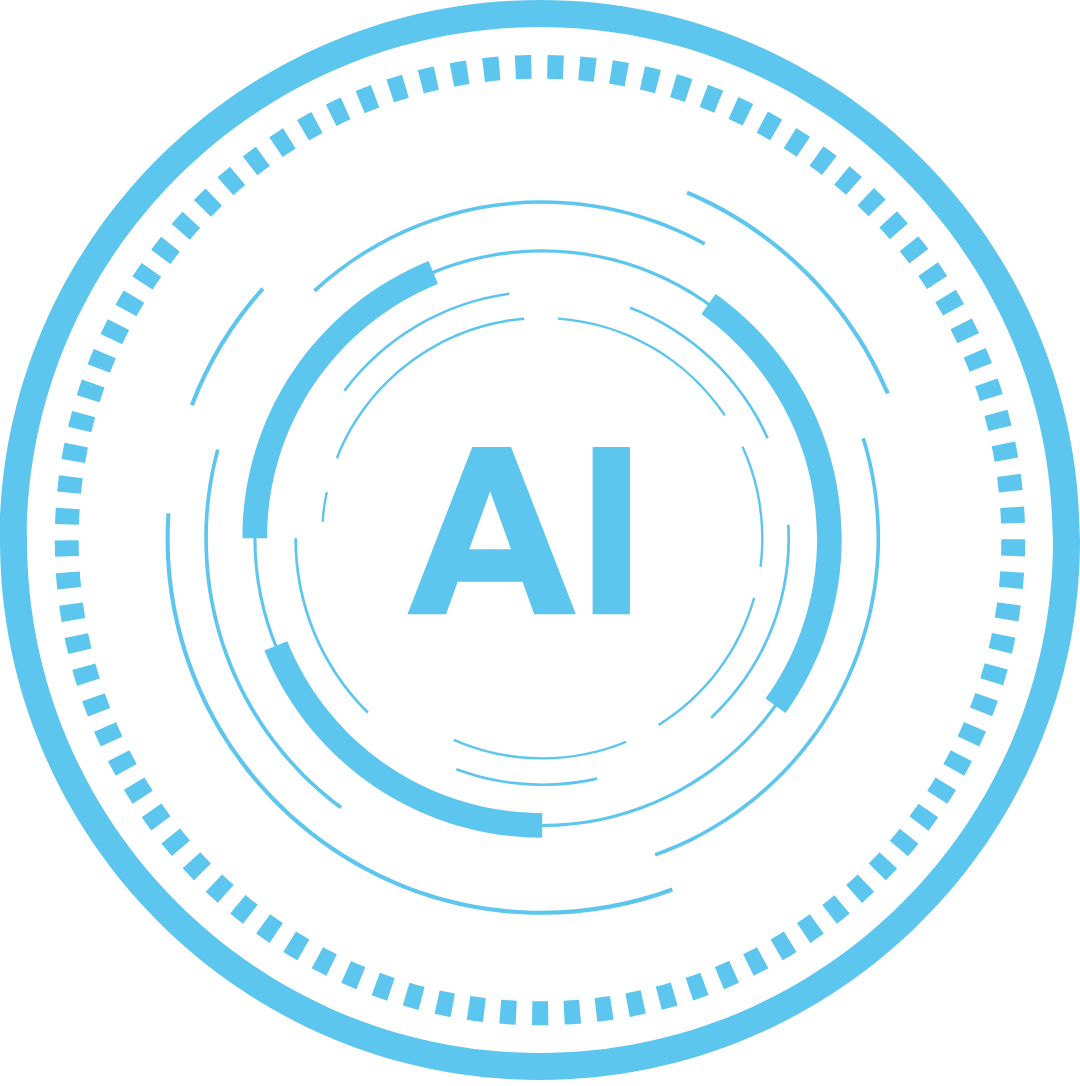
AI Robustness & Safety
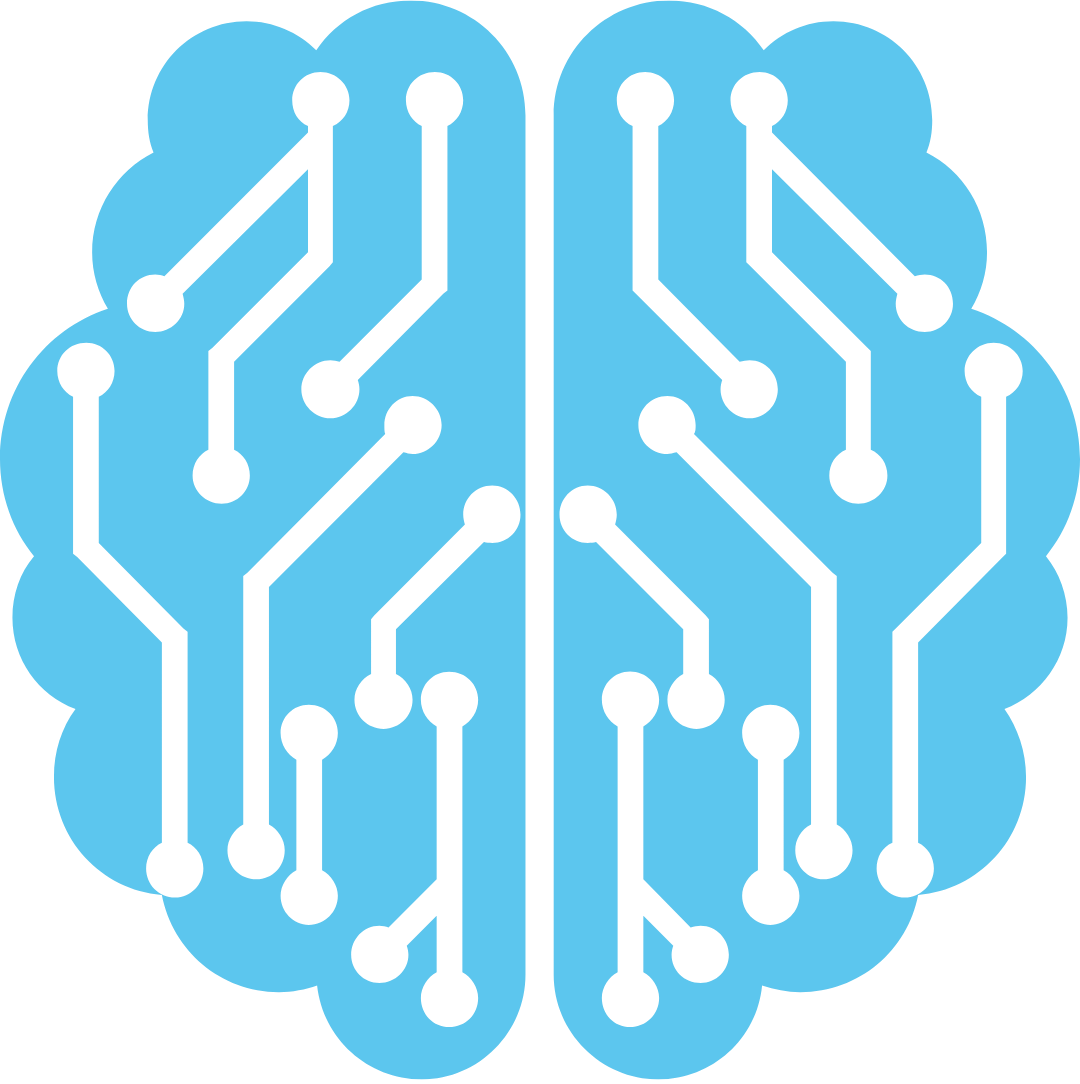
Explainable AI
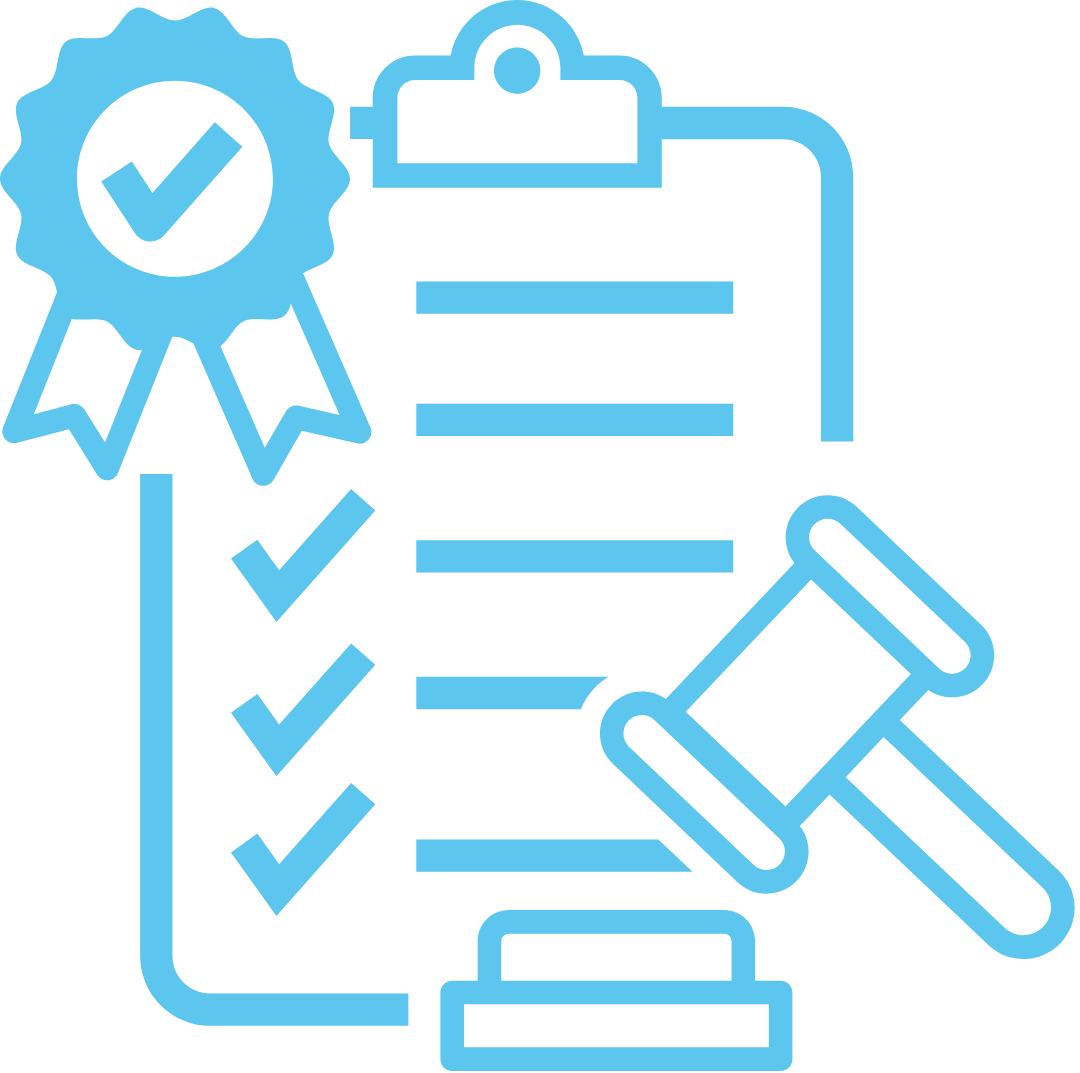
Compliance & Standards
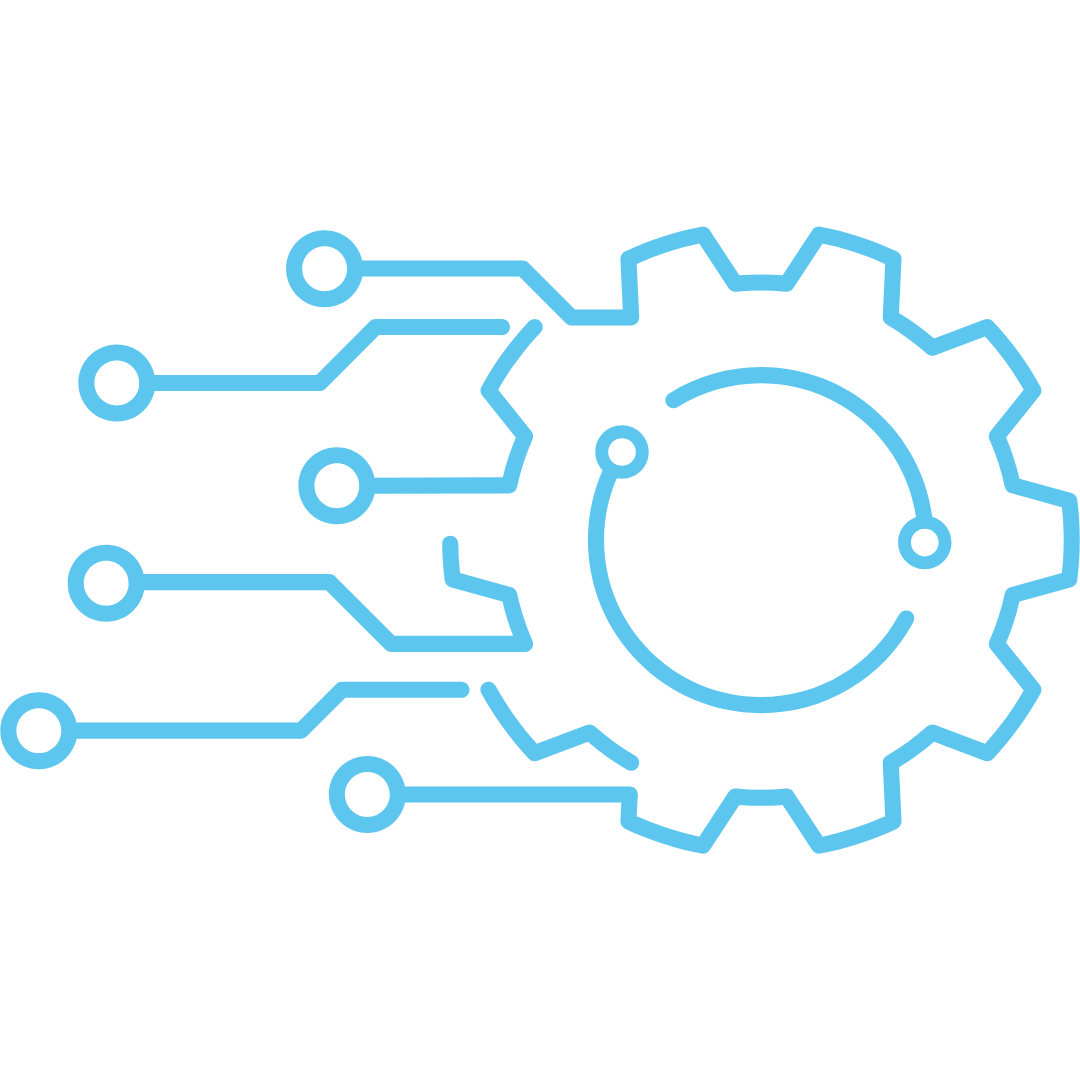
Safety Critical Applications
THE CHALLENGE: SAFE AI-BASED CRITICAL SYSTEMS
- Today’s AI allows advanced functions to run on high performance machines, but its “black‑box” decision‑making is still a challenge for automotive, rail, space and other safety‑critical applications where failure or malfunction may result in severe harm.
- Machine- and deep‑learning solutions running on high‑performance hardware enable true autonomy, but until they become explainable, traceable and verifiable, they can’t be trusted in safety-critical systems.
- Each sector enforces its own rigorous safety standards to ensure the technology used is safe (Space- ECSS, Automotive- ISO26262/ ISO21448/ ISO8800, Rail-EN 50126/8), and AI must also meet these functional safety requirements.
MAKING CERTIFIABLE AI A REALITY
Our next-generation open software platform is designed to make AI explainable, and to make systems where AI is integrated compliant with safety standards. This technology bridges the gap between cutting-edge AI capabilities and the rigorous demands for safety-crtical environments. By joining experts on AI robustness, explainable AI, functional safety and system design, and testing their solutions in safety critical applications in space, automotive and rail domains, we’re making sure we’re contribuiting to trustworthy and reliable AI.
Key activities:
SAFEXPLAIN is enabling the use of AI in safety-critical system by closing the gap between AI capabilities and functional safety requirements.
See SAFEXPLAIN technology in action
CORE DEMO
The Core Demo is built on a flexible skeleton of replaceable building blocks for Interference, Supervision or Diagnoistic components that allow it to be adapted to different secnarios. Full domain-specific demos will be available at our final event.
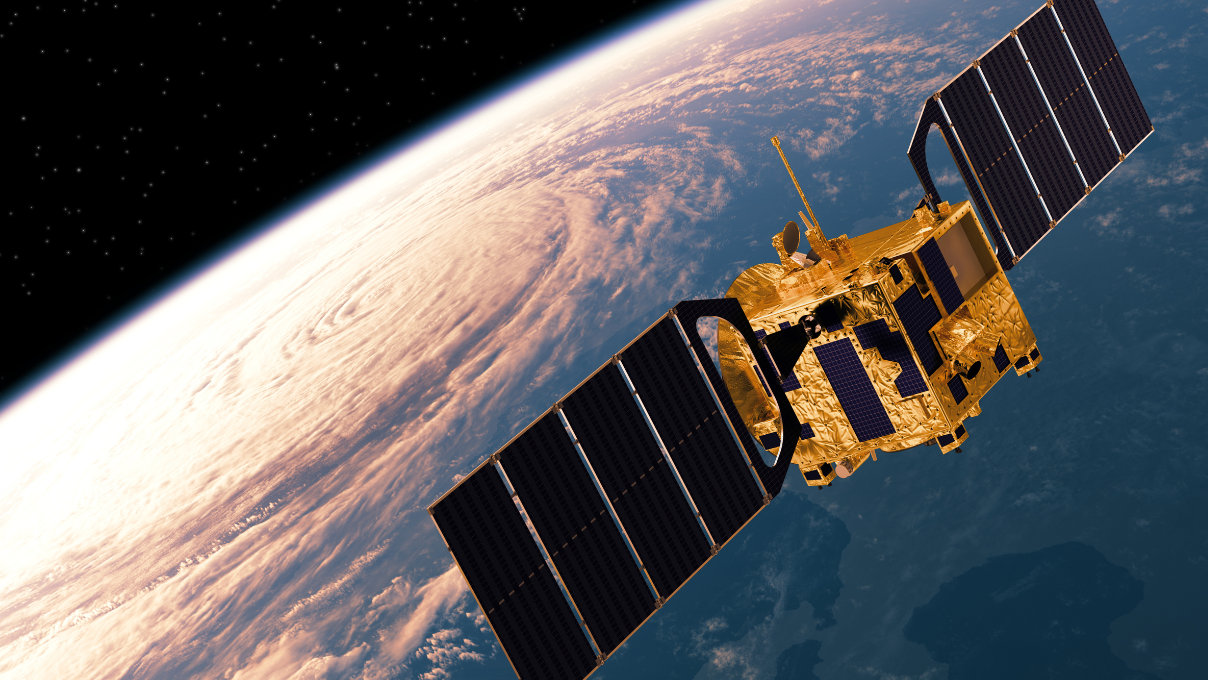
SPACE
Mission autonomy and AI to enable fully autonomous operations during space missions
Specific activities: Identify the target, estimate its pose, and monitor the agent position, to signal potential drifts, sensor faults, etc
Use of AI: Decision ensemble
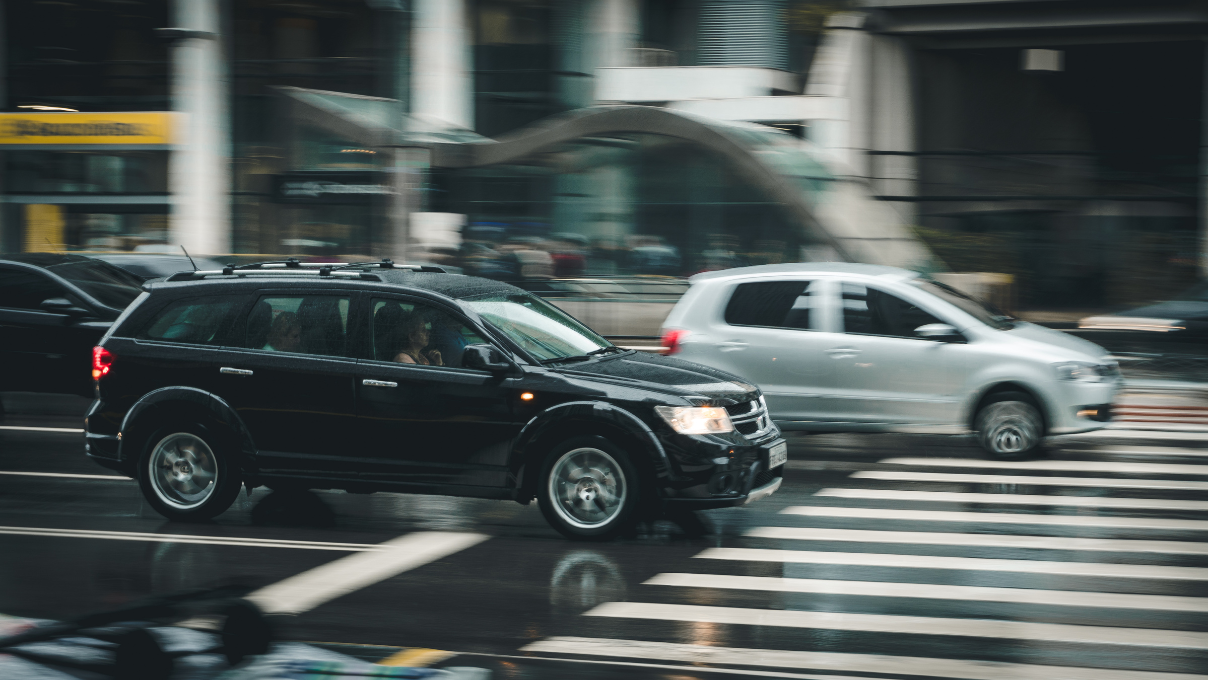
AUTOMOTIVE
Advanced methods and procedures to enable self-driving carrs to accurately detect road users and predict their trajectory
Specific activities: Validate the system’s capacity to detect pedestrians, issue warnings, and perform emergency braking
Use of AI: Decision Function (mainly visualization oriented)
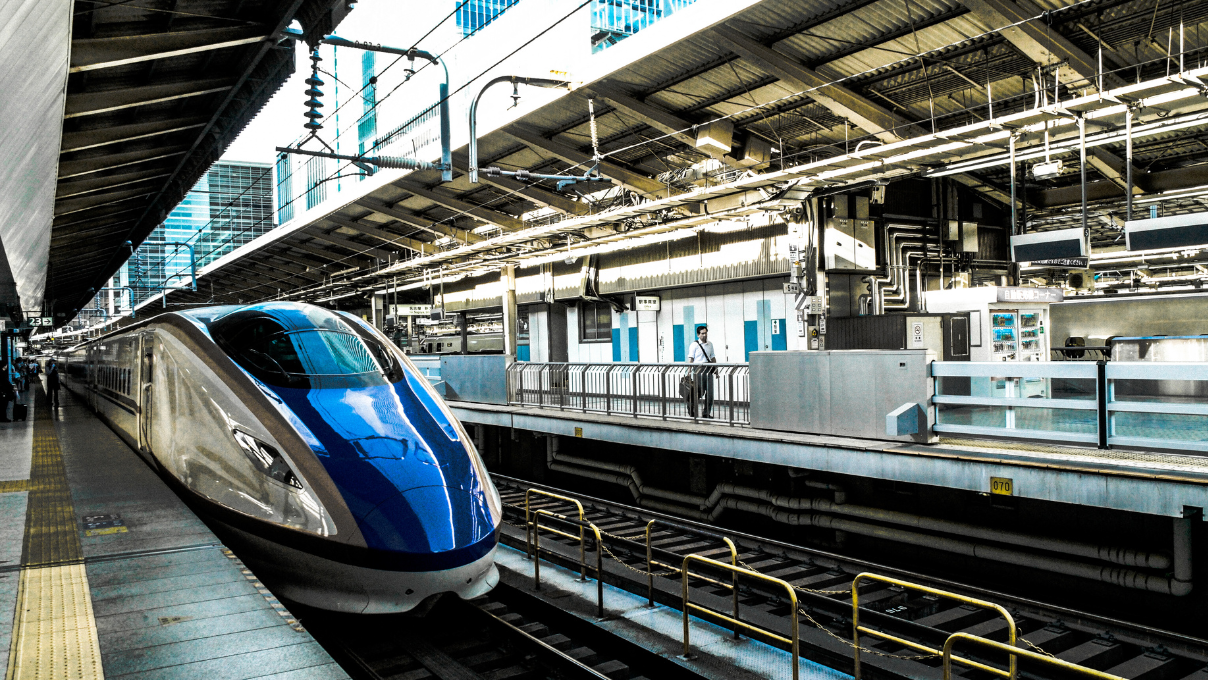
RAIL
Review of the viability of a safety architectural pattern for the completely autonomous operation of trains
Specific activities: Validate the system’s capacity to detect obstacles, issue warnings, and perform service braking
Use of AI: Data-quality and temporal consistency scores
EV community at Innovex 24 welcomes presentation by SAFEXPLAIN
SAFEXPLAIN partner, Carlo Donzella from exida development, opens EV session with key note on "Enabling the Future of EV with TrustworthyAI" June 6, 2024 marked an important opportunity for the SAFEXPLAIN project to share project results with key audiences from the...
Successful showcase of SAFEXPLAIN use cases at Trustworthy AI webinar
SAFEXPLAIN partner Enrico Mezzeti from the Barcelona Supercomputing Center joined 8 other Horizon Europe-funded projects under call HORIZON-CL4-2021-HUMAN-01-01to present the project´s work on TrustworthyAI and its implications for its use cases. The nine projects,...
A Tale of Machine Learning Process Models at Automotive SPIN Italia
Carlo Donzella from exida development presents at the Automotive SPIN Italia 22º Workshop on Automotive Software & System SAFEXPLAIN partner Carlo Donzella, from exida development, presented at the Automotive SPIN Italia 22º Workshop on Automotive Software &...
Expert Panel on AI-Enabled Software Development Tools: Exploring Safety-Critical Applications
Location: Lisbon, Portugal Participants: Ikerlan's Jon Pérez and other industry experts Date: June 15, 2023 SAFEXPLAIN partner, Jon Pérez from Ikerlan was an invited speaker at the ADA-Europe International Conference on Reliable Software Technologies (AEiC) in...
SAFEXPLAIN’s Presentation “Efficient Diverse Redundant DNNs for Autonomous Driving” Accepted for COMPSAC 2023
SAFEXPLAIN presented at the Conference on Computers, Software, and Applications (COMPSAC) 2023. The paper, 'Efficient Diverse Redundant DNNs for Autonomous Driving' was accepted for publication at the IEEE Computer Society Signature Conference on Computers, Software,...
Standardizing the Probabilistic Sources of Uncertainty for the sake of Safety Deep Learning
The Safexplain team from the CAOS research group of the Barcelona Supercomputing Center (BSC-CNS) presented their latest research at the AAAI's Workshop on Artificial Intelligence Safety of the AAAI 2023, held on February 14, 2023, in Washington D.C. The team's...