
Railway Case Study
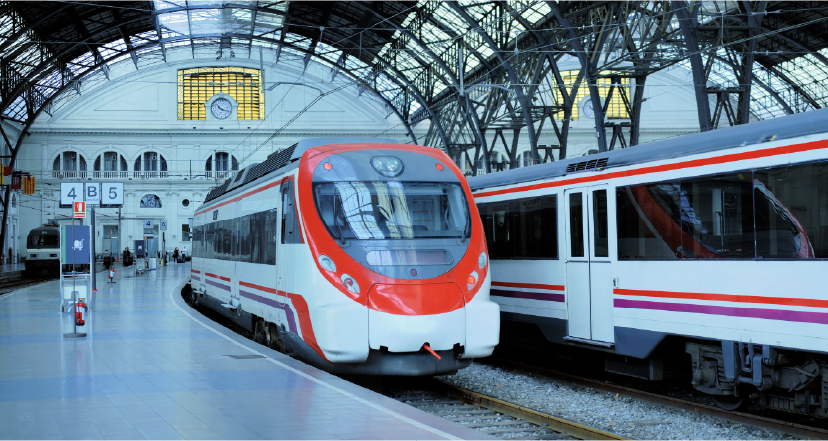
Driverless trains are experiencing an unprecedented growth. Current forecasts indicate that by 2025 there will be over 2300 km of fully automated metro lines. However, driverless trains have not yet been deployed for long distance high-speed and regional passenger goods and trains. Train speed and interaction with environmental factors limit the expansion of automated metro trains technology into long distance rail networks.
The SAFEXPLAIN railway case study will check the viability of a safety architectural pattern for the completely autonomous operation of trains (Automatic Train Operation, ATO). The project will employ intelligent Deep Learning (DL)-based solutions, including artificial vision elements, to detect and locate people and obstacles on the track and in the way of the train doors and to estimate their position to ensure the train does not collide with obstacles or injure passengers. Safety-related software elements and DL software elements implement the safety function that allow trains to make safe and optimal decisions in real-time.
Moving from automated trains to autonomous trains will provide a range of benefits including:
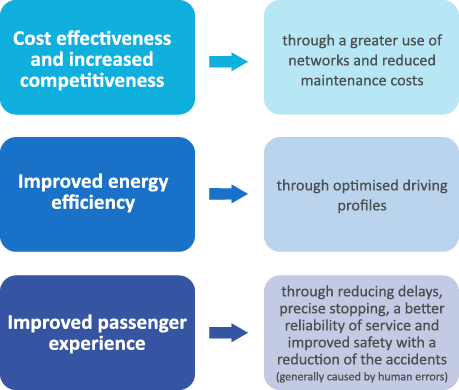
Making safe and optimal decisions in real-time
Challenge:
The SAFEXPLAIN project seeks to close the gap between Functional Safety Requirements and the nature of Deep Learning solutions. Functional Safety systems need deterministic, verifiable and pass/fail test-based software solutions, however, DL-based solutions currently lack explainability and traceability, and lack robustness, security and fault-tolerance.
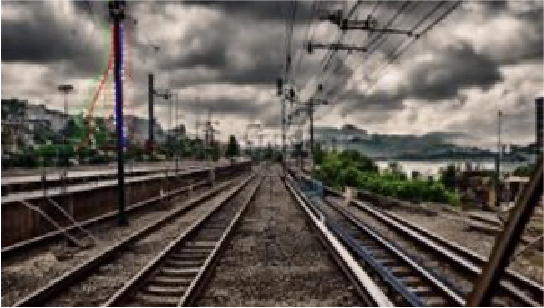
Figure 2a : Image derived from DL artificial software elements that serve as sensors.
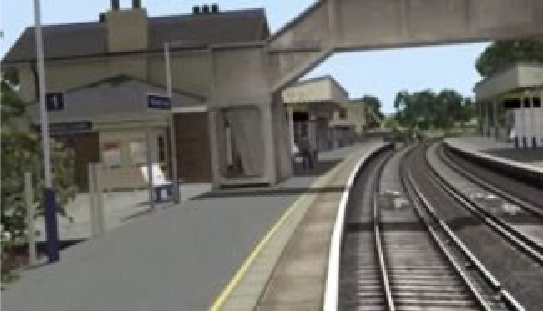
Figure 2b: Image derived from DL artificial software elements that serve as sensors.
Role of explainability AI: AI explainability may help during the process of safety system certification by proposing evidence that helps show certification authorities that AI-based sensors are properly trained and work as expected. AI explainability may also help by adding diagnosis capabilities to the safety system to implement redundant systems with diagnosis capabilities during safety system operation.
Read more about our progress in the railway case study:
- Gauging requirements and testing models for Space, Automotive and Railway Case Studies
- Taking Automatic Train Operation further: implementing safety functions to minimize risk
- Four Ways of Testing how to Minimize Risk of Injury in Automatic Train Operation
- Integrating the Railway Case Study into the Reference Safety Architecture Pattern
- D5.1 Case study stubbing and early assessment of case study porting