SAFEXPLAIN: From Vision to Reality
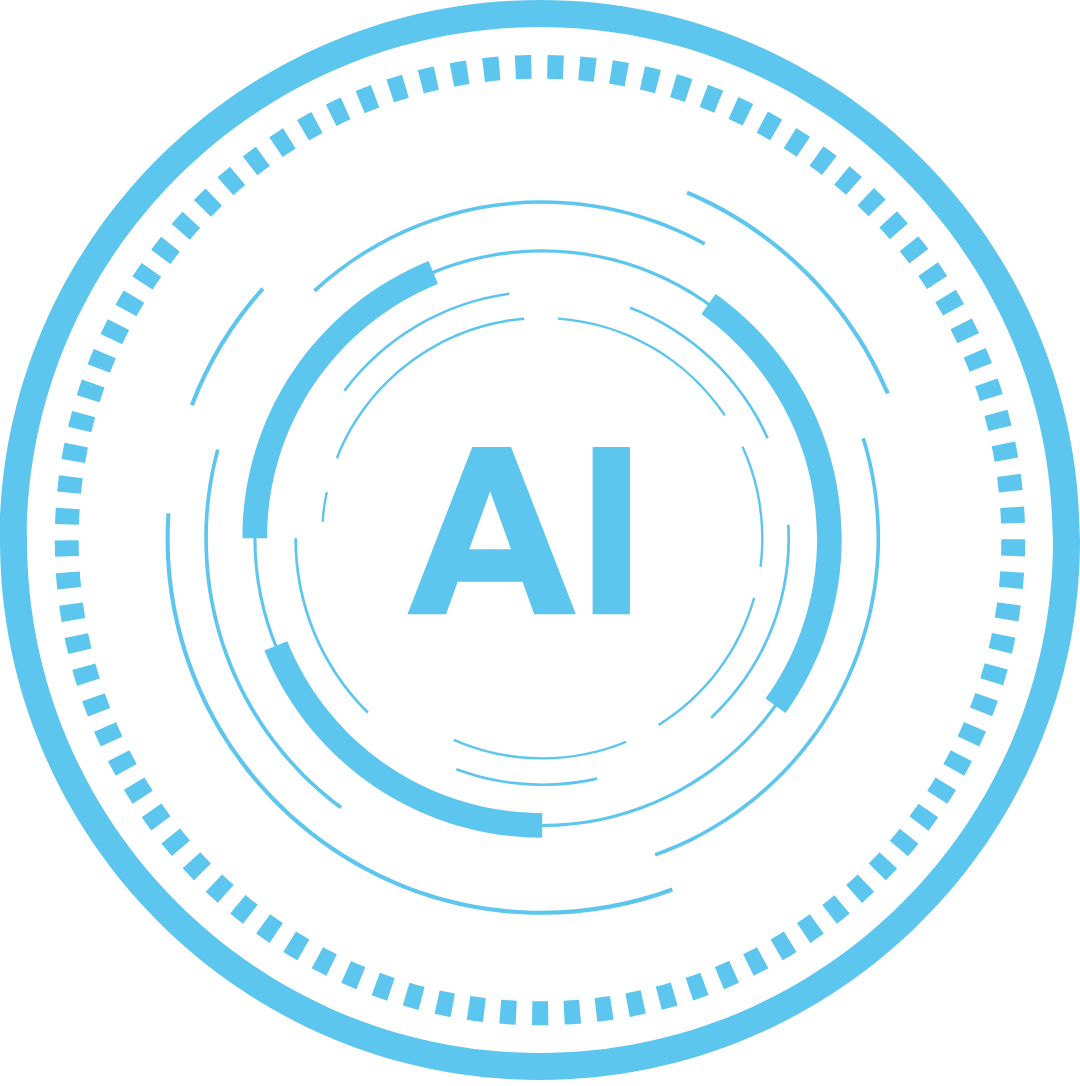
AI Robustness & Safety
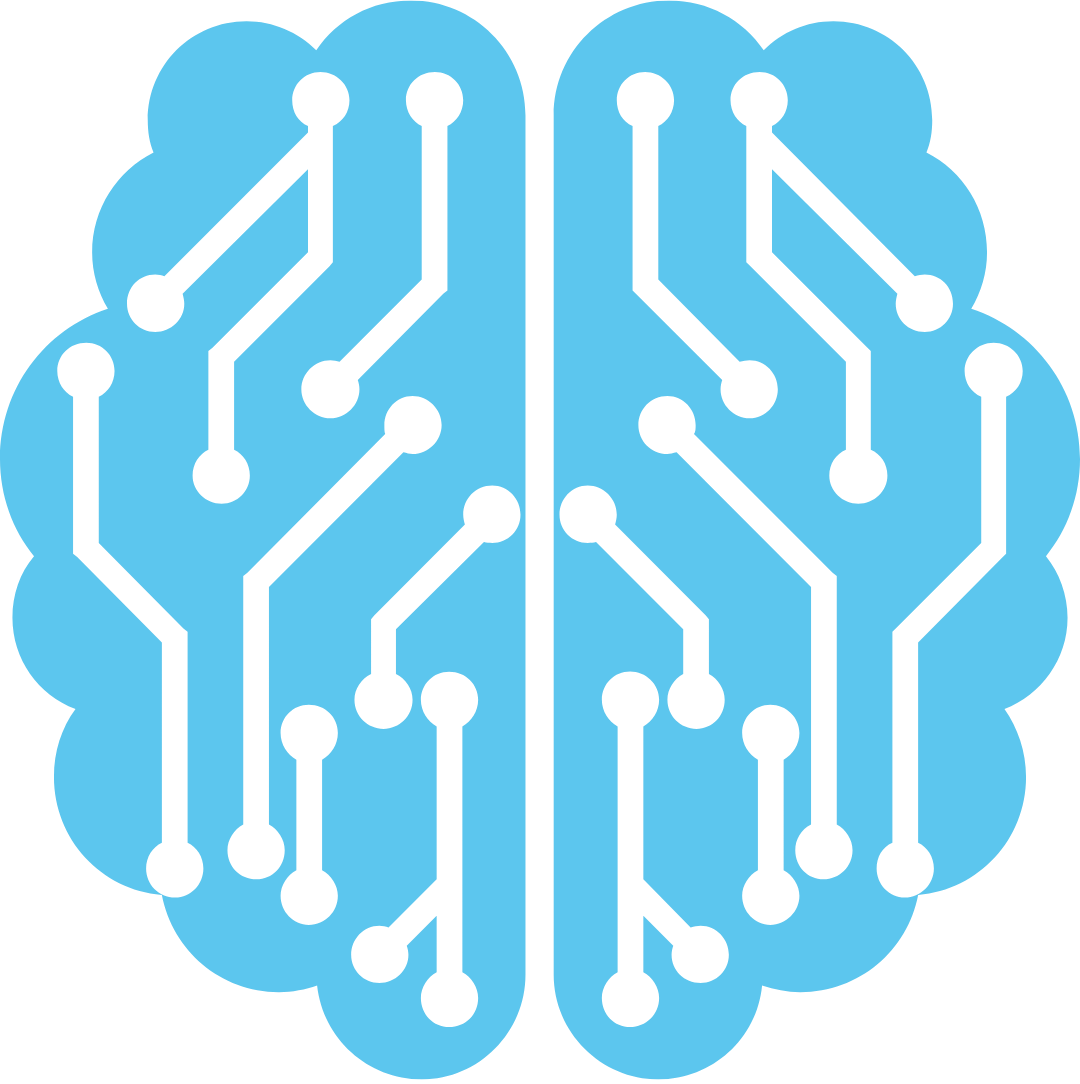
Explainable AI
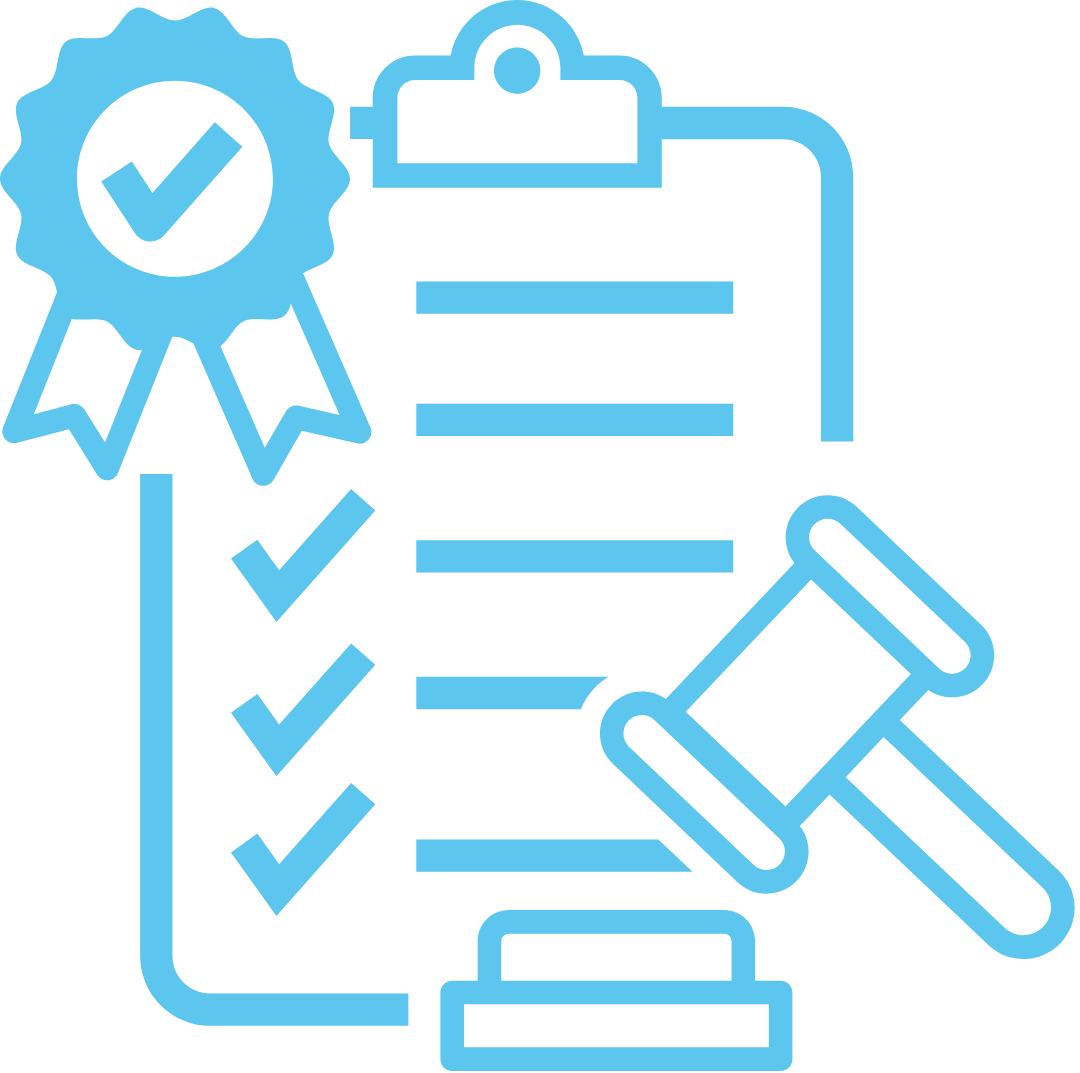
Compliance & Standards
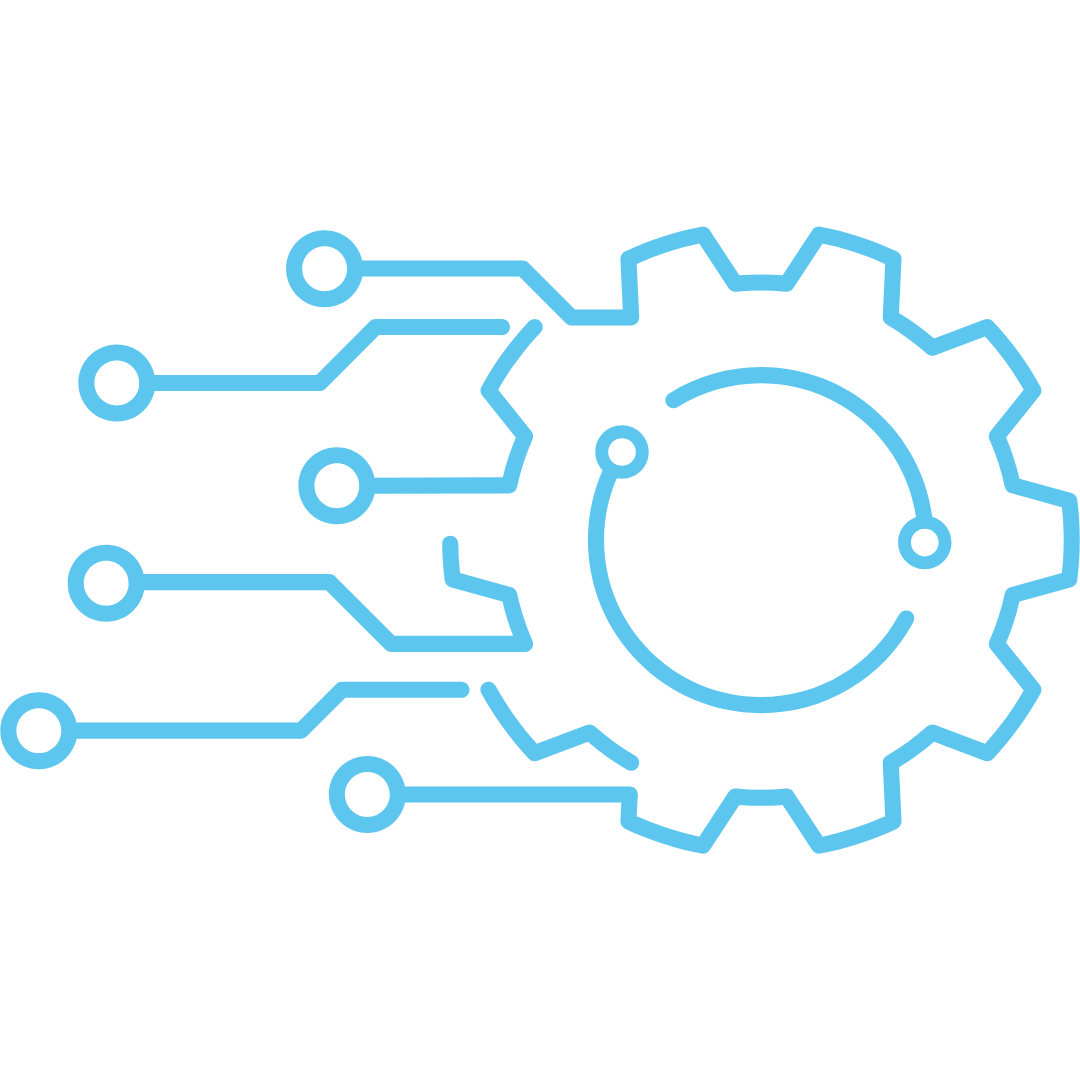
Safety Critical Applications
THE CHALLENGE: SAFE AI-BASED CRITICAL SYSTEMS
- Today’s AI allows advanced functions to run on high performance machines, but its “black‑box” decision‑making is still a challenge for automotive, rail, space and other safety‑critical applications where failure or malfunction may result in severe harm.
- Machine- and deep‑learning solutions running on high‑performance hardware enable true autonomy, but until they become explainable, traceable and verifiable, they can’t be trusted in safety-critical systems.
- Each sector enforces its own rigorous safety standards to ensure the technology used is safe (Space- ECSS, Automotive- ISO26262/ ISO21448/ ISO8800, Rail-EN 50126/8), and AI must also meet these functional safety requirements.
MAKING CERTIFIABLE AI A REALITY
Our next-generation open software platform is designed to make AI explainable, and to make systems where AI is integrated compliant with safety standards. This technology bridges the gap between cutting-edge AI capabilities and the rigorous demands for safety-crtical environments. By joining experts on AI robustness, explainable AI, functional safety and system design, and testing their solutions in safety critical applications in space, automotive and rail domains, we’re making sure we’re contribuiting to trustworthy and reliable AI.
Key activities:
SAFEXPLAIN is enabling the use of AI in safety-critical system by closing the gap between AI capabilities and functional safety requirements.
See SAFEXPLAIN technology in action
CORE DEMO
The Core Demo is built on a flexible skeleton of replaceable building blocks for Interference, Supervision or Diagnoistic components that allow it to be adapted to different secnarios. Full domain-specific demos will be available at our final event.
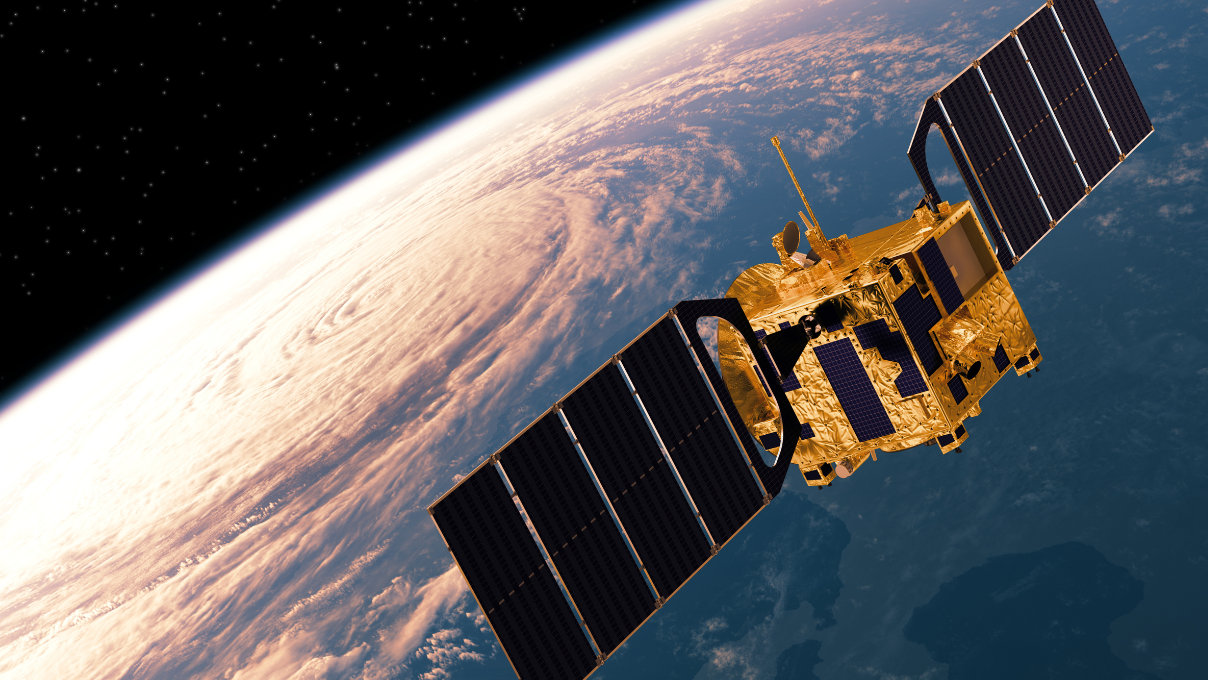
SPACE
Mission autonomy and AI to enable fully autonomous operations during space missions
Specific activities: Identify the target, estimate its pose, and monitor the agent position, to signal potential drifts, sensor faults, etc
Use of AI: Decision ensemble
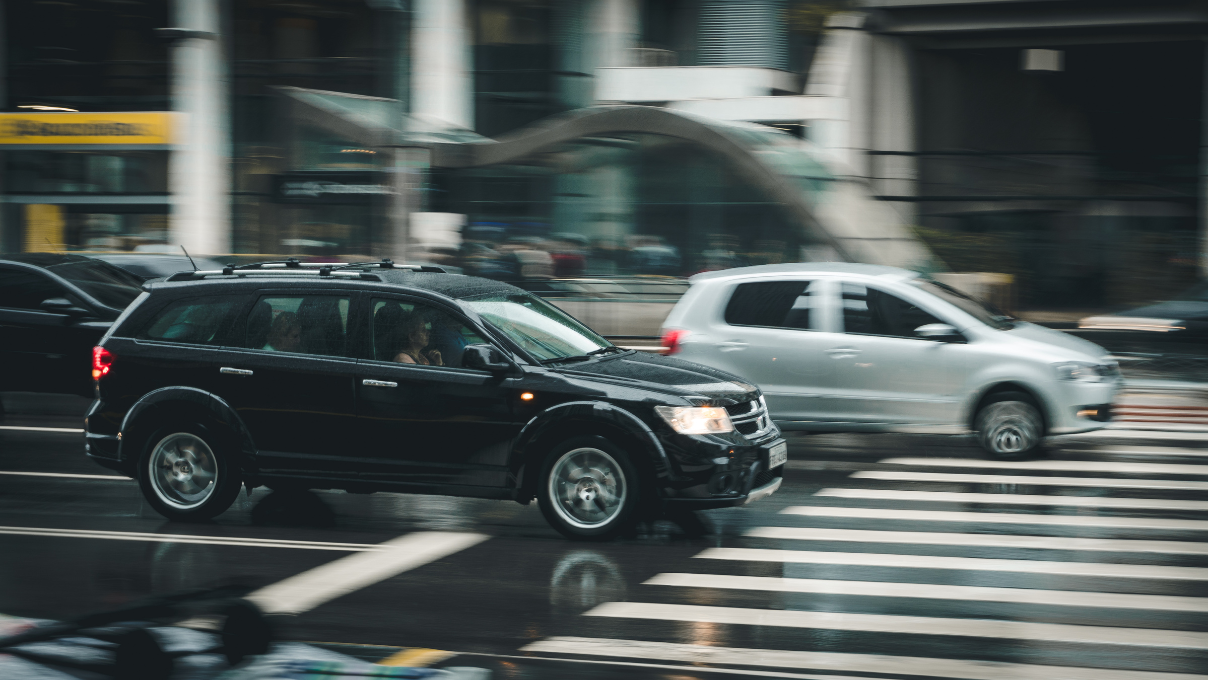
AUTOMOTIVE
Advanced methods and procedures to enable self-driving carrs to accurately detect road users and predict their trajectory
Specific activities: Validate the system’s capacity to detect pedestrians, issue warnings, and perform emergency braking
Use of AI: Decision Function (mainly visualization oriented)
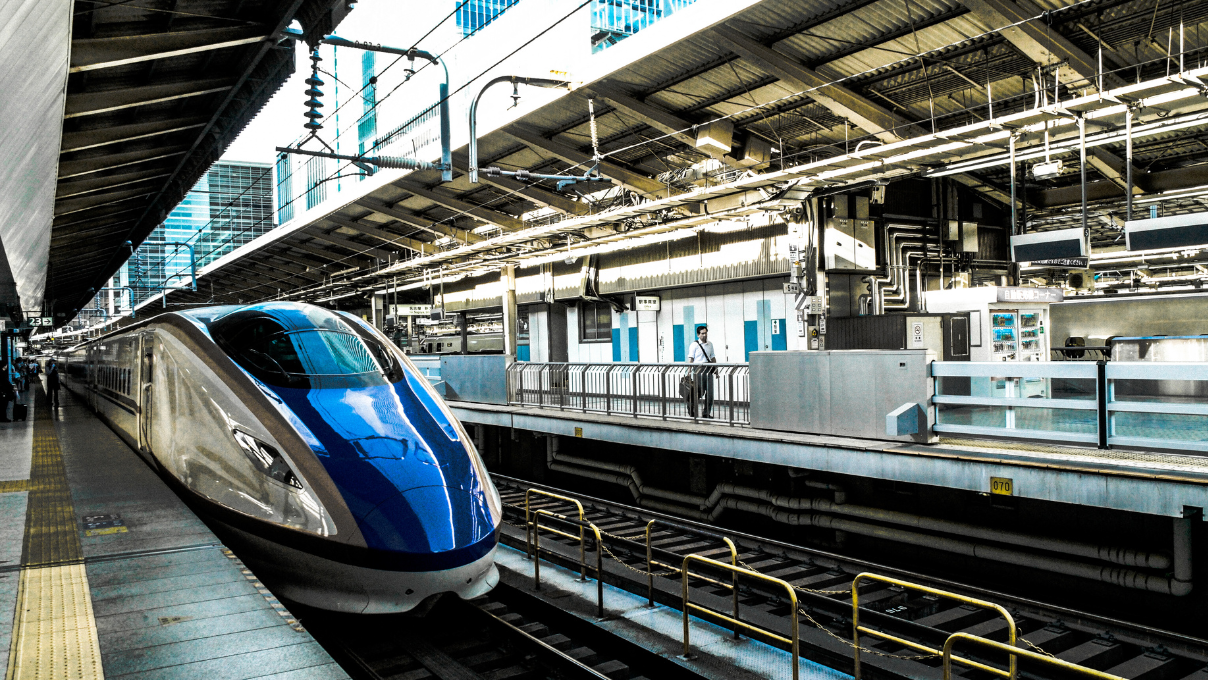
RAIL
Review of the viability of a safety architectural pattern for the completely autonomous operation of trains
Specific activities: Validate the system’s capacity to detect obstacles, issue warnings, and perform service braking
Use of AI: Data-quality and temporal consistency scores
Safety for AI-Based Systems
As part of SAFEXPLAIN, Exida has contributed a methodology related to a verification and validation (V&V) strategy of AI-based components in safety-critical systems. The approach combines the two standards ISO 21448 (also known as SOTIF) and ISO 26262 to address...
PRESS RELEASE: SAFEXPLAIN Unveils Core Demo: A Step Further Toward Safe and Explainable AI in Critical Systems
Barcelona, 03 July 2025 The SAFEXPLAIN project has just publicly unveiled its Core Demo, offering a concrete look at how its open software platform can bring safe, explainable and certifiable AI to critical domains like space, automotive and rail. Showcased in a...
Core Demo Webinar- Making certifiable AI a reality for critical systems
Now available!
The project’s Core Demo is a small-scale, modular demonstrator that highlights the platform’s key technologies and showcases how AI/ML components can be safely integrated into critical systems.
Two SAFEXPLAIN Papers Accepted at DSD 2025
SAFEXPLAIN partners will share key projects results at the 2025 28th Euromicro Conference Series on Digital System Design (DSD). Two papers have been accepted to the conference proceedings. These publications describe the consolidation of key results emerging from...
WEBINAR- Making certifiable AI a reality for critical systems: CORE DEMO
Register here Get a sneak peek about what the core demo is about
SAFEXPLAIN Results in Action: the integrated SW Platform- Presentation & booth at ASPIN
SAFEXPLAIN representatives will present at the 23° WORKSHOP on AUTOMOTIVE SOFTWARE & SYSTEMS hosted by Automotive SPIN Italia on 29 May 2025. As part of the session on Functional Safety, Carlo Donzella from exida development and Enrico Mezzetti from the Barcelona...