SAFEXPLAIN: From Vision to Reality
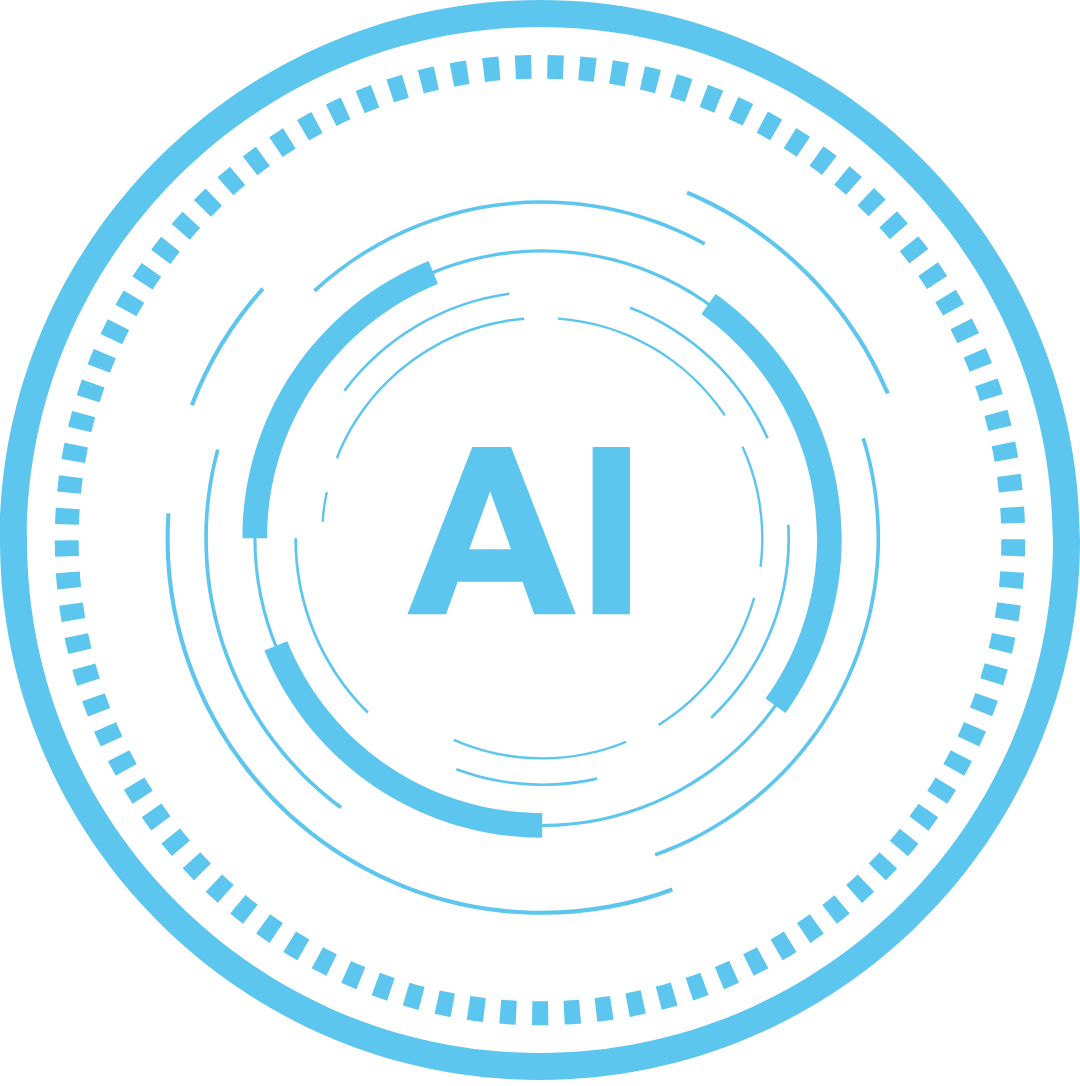
AI Robustness & Safety
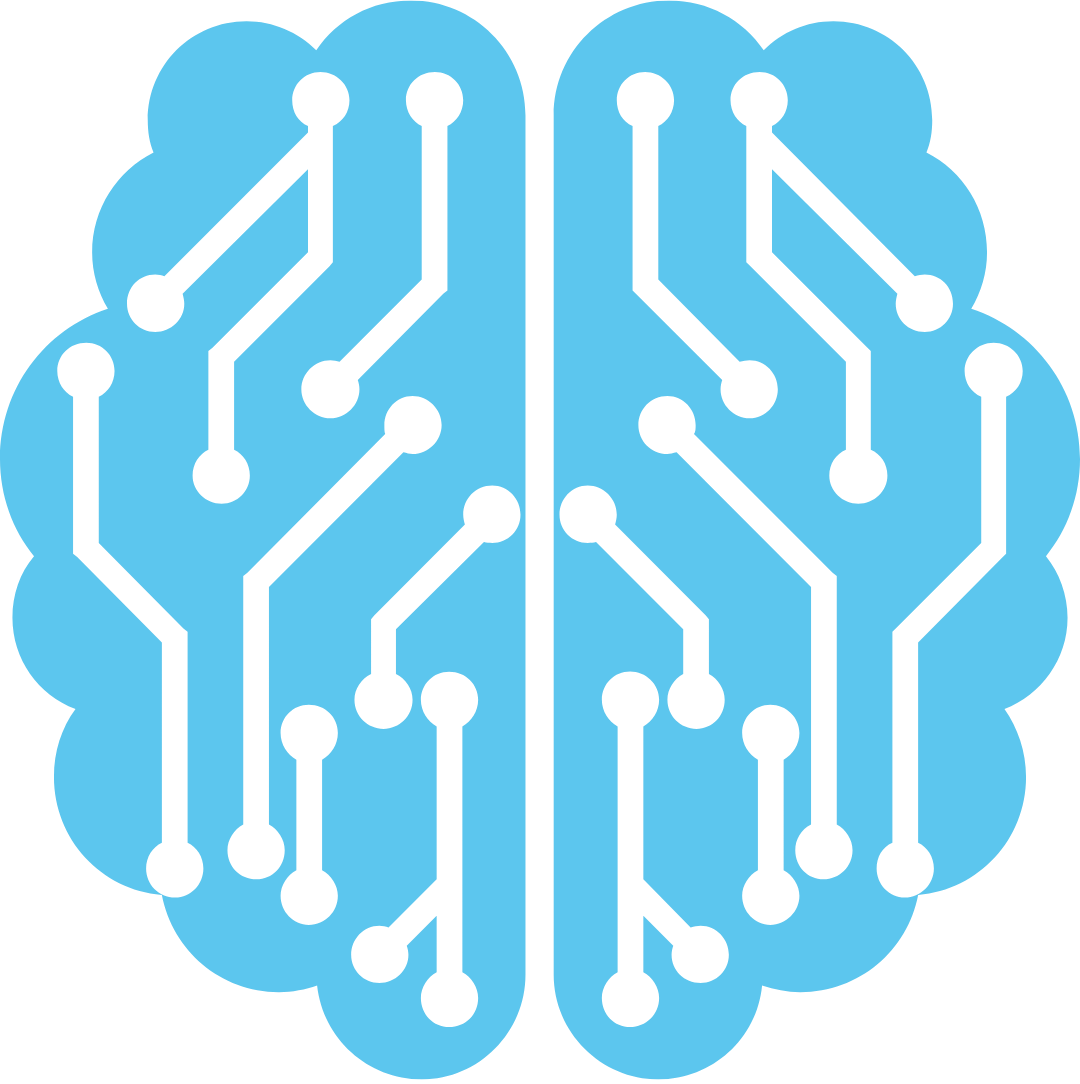
Explainable AI
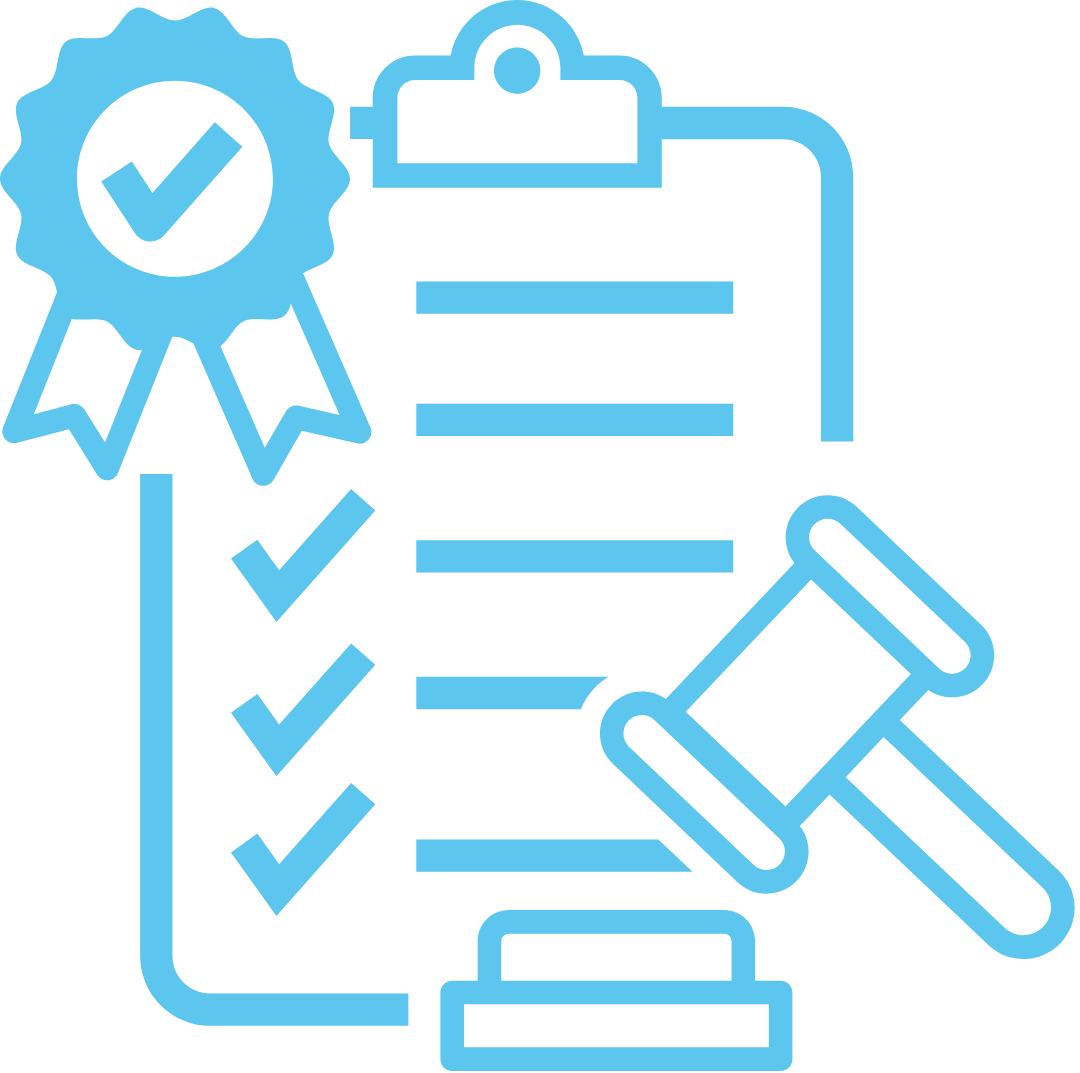
Compliance & Standards
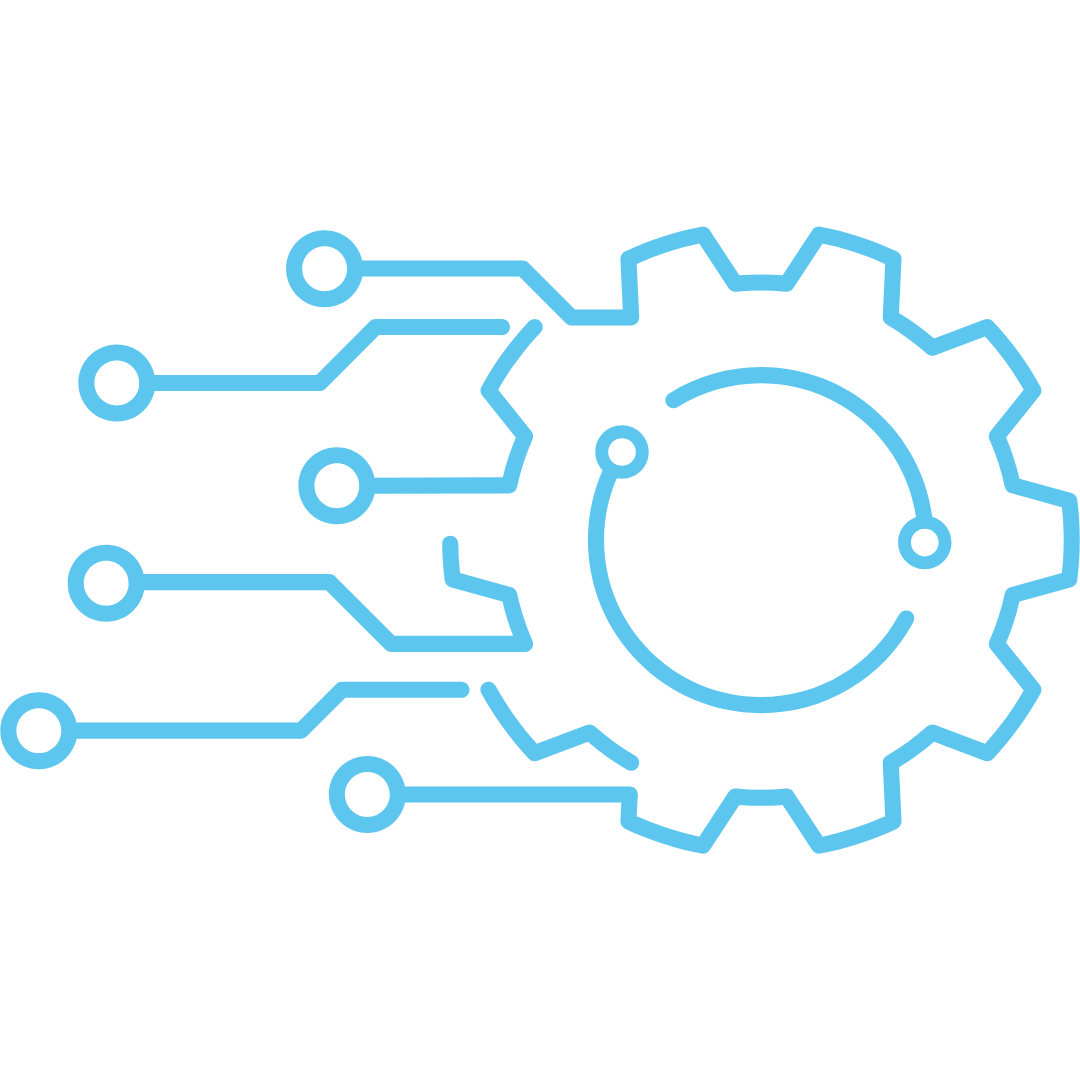
Safety Critical Applications
THE CHALLENGE: SAFE AI-BASED CRITICAL SYSTEMS
- Today’s AI allows advanced functions to run on high performance machines, but its “black‑box” decision‑making is still a challenge for automotive, rail, space and other safety‑critical applications where failure or malfunction may result in severe harm.
- Machine- and deep‑learning solutions running on high‑performance hardware enable true autonomy, but until they become explainable, traceable and verifiable, they can’t be trusted in safety-critical systems.
- Each sector enforces its own rigorous safety standards to ensure the technology used is safe (Space- ECSS, Automotive- ISO26262/ ISO21448/ ISO8800, Rail-EN 50126/8), and AI must also meet these functional safety requirements.
MAKING CERTIFIABLE AI A REALITY
Our next-generation open software platform is designed to make AI explainable, and to make systems where AI is integrated compliant with safety standards. This technology bridges the gap between cutting-edge AI capabilities and the rigorous demands for safety-crtical environments. By joining experts on AI robustness, explainable AI, functional safety and system design, and testing their solutions in safety critical applications in space, automotive and rail domains, we’re making sure we’re contribuiting to trustworthy and reliable AI.
Key activities:
SAFEXPLAIN is enabling the use of AI in safety-critical system by closing the gap between AI capabilities and functional safety requirements.
See SAFEXPLAIN technology in action
CORE DEMO
The Core Demo is built on a flexible skeleton of replaceable building blocks for Interference, Supervision or Diagnoistic components that allow it to be adapted to different secnarios. Full domain-specific demos will be available at our final event.
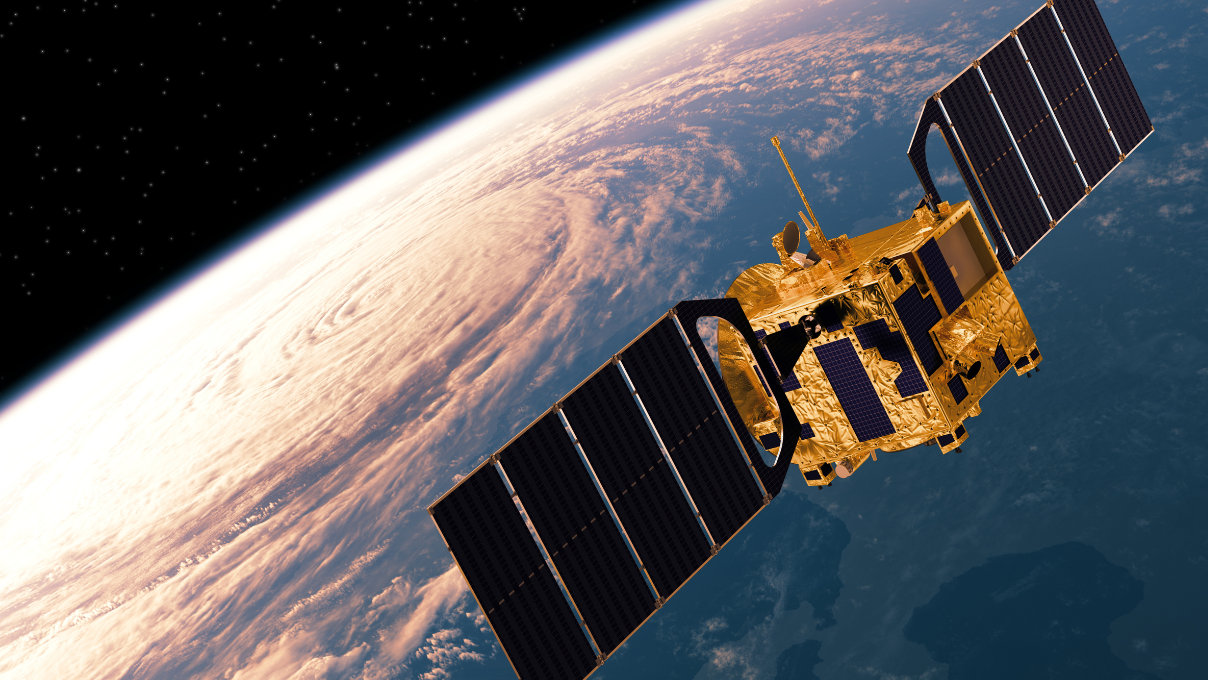
SPACE
Mission autonomy and AI to enable fully autonomous operations during space missions
Specific activities: Identify the target, estimate its pose, and monitor the agent position, to signal potential drifts, sensor faults, etc
Use of AI: Decision ensemble
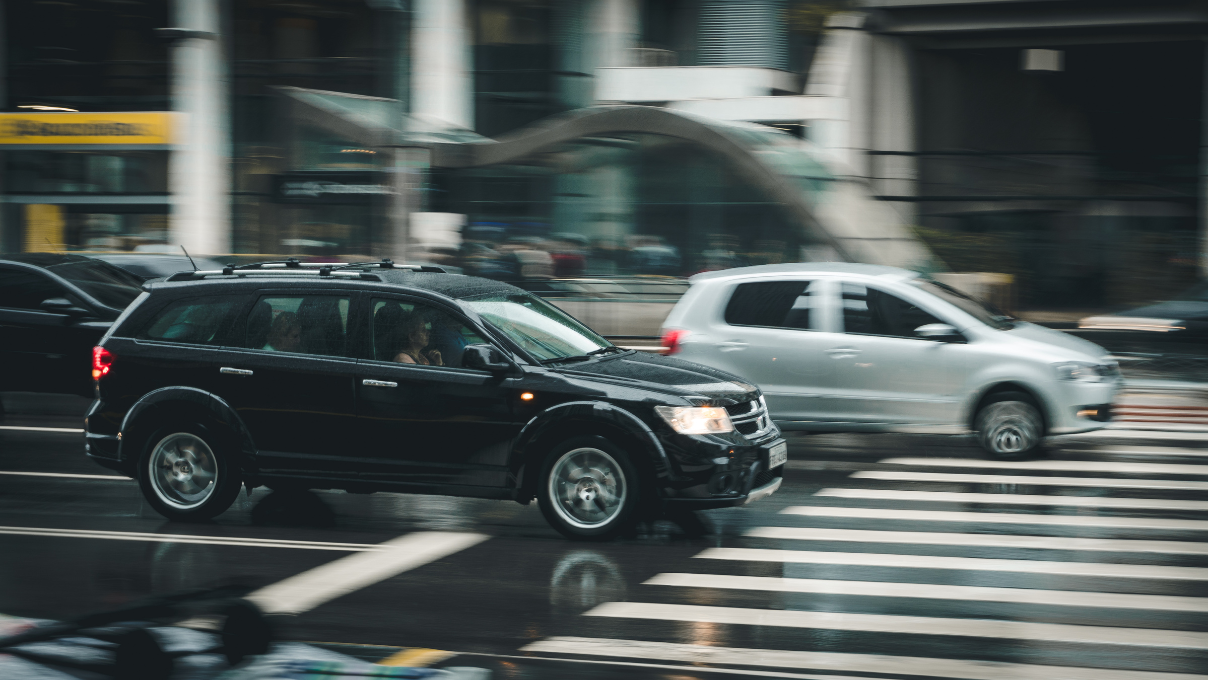
AUTOMOTIVE
Advanced methods and procedures to enable self-driving carrs to accurately detect road users and predict their trajectory
Specific activities: Validate the system’s capacity to detect pedestrians, issue warnings, and perform emergency braking
Use of AI: Decision Function (mainly visualization oriented)
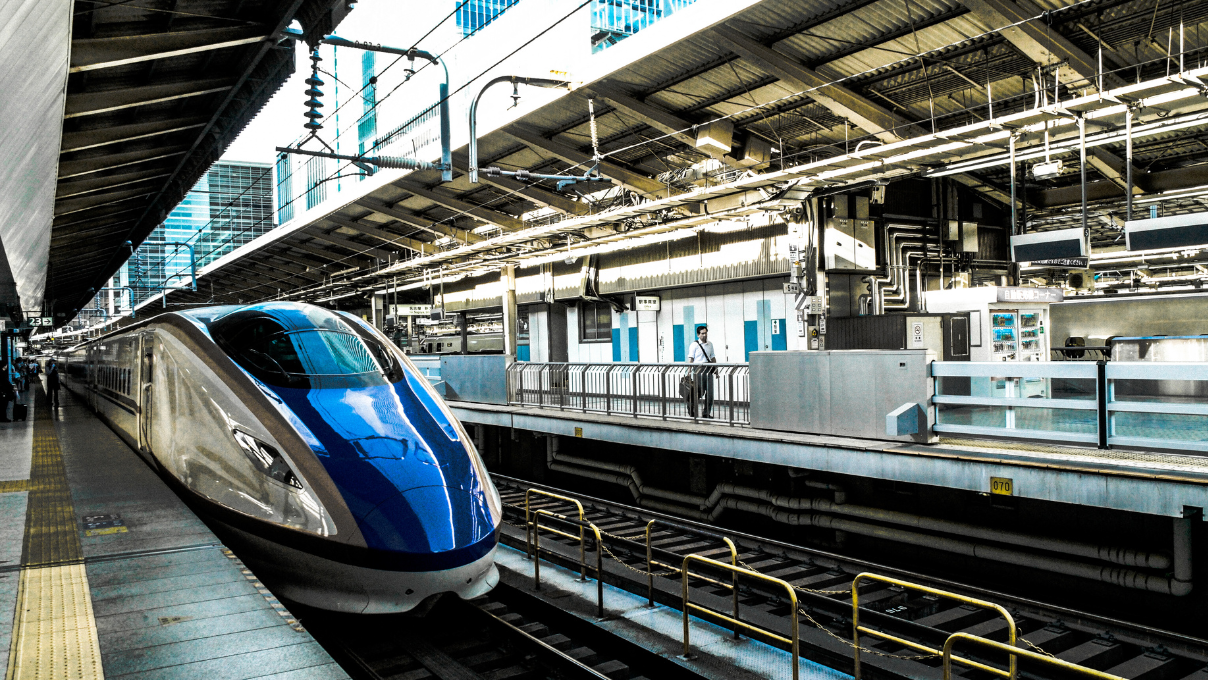
RAIL
Review of the viability of a safety architectural pattern for the completely autonomous operation of trains
Specific activities: Validate the system’s capacity to detect obstacles, issue warnings, and perform service braking
Use of AI: Data-quality and temporal consistency scores
Tackling Uncertainty in AI for Safer Autonomous Systems
Within the SAFEXPLAIN project, members of the Research Institues of Sweden (RISE) team have been evaluating and implementing components and architectures for making AI dependable when utilised within safety-critical autonomous systems. To contribute to dependability...
SAFEXPLAIN shares its safety critical solutions with aerospace industry representatives
On 12 May 2025, the SAFEXPLAIN consortium presented its latest results to representatives of several aerospace and embedded system industries including Airbus DS; BrainChip, the European Space Agency (ESA), Gaisler, and Klepsydra, showcasing major strides in making AI...
SAFEXPLAIN Update: Building Trustworthy AI for Safer Roads
For enhanced safety in AI-based systems in the railway domain, the SAFEXPLAIN project has been working to integrate cutting-edge simulation technologies with robust communication frameworks. Learn more about how we’re integrating Unreal Engine (UE) 5 with Robot Operating System 2 (ROS 2) to generate dynamic, interactive simulations that accurately replicate real-world railway scenarios.
40th ACM/SIGAPP symposium on Applied Computing
On 4 April 2025, Sergi Vilardell from the Barcelona Supercomputing Center will present "Probabilistic Timing Estimates in Scenarios Under Testing Constraints " as part of the Conference track on System Software and Security EMBS, Embedded Systems. The 40th ACM/SIGAPP...
Managing Sources of Uncertainty in Utilizing AI in Development and Deployment of Safety-Critical Autonomous Systems- Presentation at SAML ’25
SAFEXPLAIN project partner RISE presented as part of the 4th International Workshop on Software Architecture and Machine Learning. On 1 April 2025, Robert Lowe presented "Managing Sources of Uncertainty in Utilizing AI in Development and Deployment of Safety-Critical...
Future-Ready On-Demand Solutions with AI, Data, and Robotics
SAFEXPLAIN project coordinator, Jaume Abella, represented the project as part of the first day of the “Future-Ready: On-Demand Solutions with AI, Data, and Robotics” event. The TrustworthyAI Cluster held a Birds of a Feather workshop on main innovations and future challenges on 18 Feb 2025 at 10:30.